The following Insight is a featured article from WCG’s 2025 Trends & Insights Report. If you would like to read more insights from this report, please click here.
In precision medicine, particularly in oncology, artificial intelligence (AI) is transforming patient care through predictive biomarker matching, which enables personalized treatment approaches that surpass traditional one-size-fits-all methodologies. Biomarkers, which are signature molecules that reflect biological states, have long been used to guide treatment decisions by predicting disease progression, patient response, and potential therapeutic efficacy. Biomarker-informed therapies have demonstrated success, with treatment response rates increasing from 20% to approximately 42% when biomarkers are used, according to data from the Personalized Medicine Coalition. However, challenges in data integration and the complexities of disease heterogeneity limit the widespread clinical adoption of biomarker-driven approaches.
AI, specifically machine learning (ML), is proving to be pivotal in addressing these challenges. Unlike traditional biomarker methods that focus on individual traits, AI enables researchers to interpret intricate patterns across thousands of biological data points, creating a more holistic understanding of disease biology. For example, sophisticated ML algorithms can integrate diverse datasets, including genomic information, proteomics, and clinical trial data, allowing clinicians to develop dynamic, personalized treatment strategies. This data harmonization provides a comprehensive view of patient biological profiles, enhancing the precision of treatment selection.
In practice, AI aids in multiple key areas of biomarker matching: data integration and management, predictive modeling, and dynamic biomarker tracking. Integrating data from various sources, such as electronic health records (EHRs), specialty labs and genomic databases, is crucial for effective biomarker discovery. Predictive analytics then enable clinicians to identify patients most likely to benefit from specific therapies, minimizing the trial-and-error approach that can characterize traditional treatments. Moreover, as biomarkers can evolve over time, AI’s continuous tracking capabilities allow for real-time adjustments, ensuring ongoing treatment relevancy.
However, as AI-driven biomarker matching advances, ethical considerations regarding patient data privacy and algorithmic accuracy and transparency remain essential. Promoting ethical standards and transparency in AI applications fosters trust and ensures that technology translates into meaningful patient benefits. As AI continues to redefine biomarker matching in 2025 and beyond, it holds the potential to revolutionize clinical trial success rates, improve patient outcomes, and ultimately reduce healthcare costs across therapeutic areas.
Related Insights:
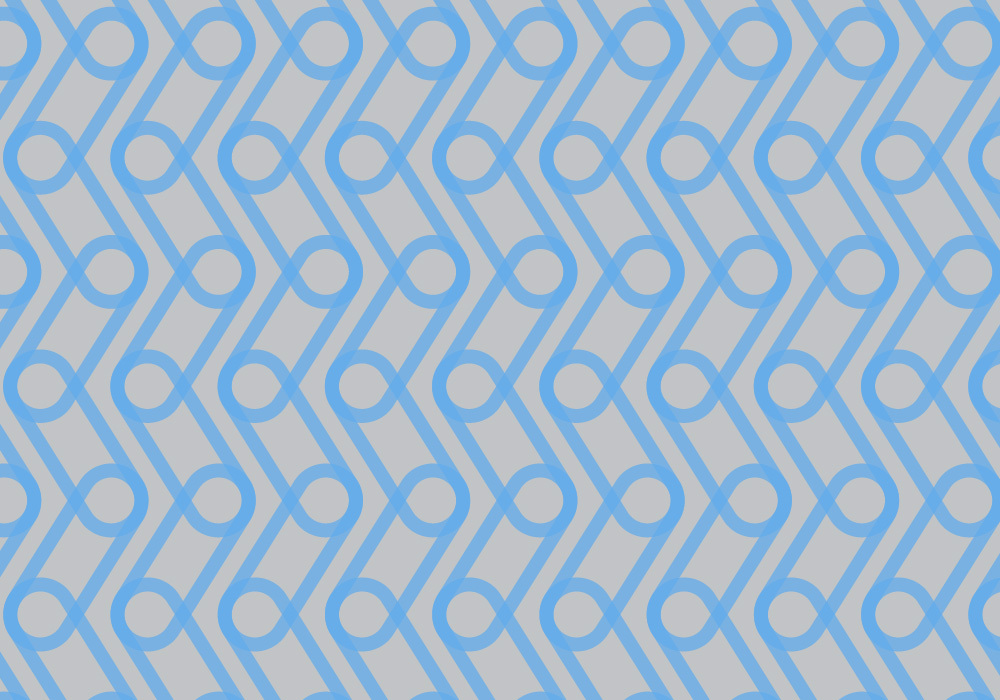
Ethical Review & AI in Clinical Trials
PodcastsLearn more about our Trends and Insights Report for 2025
Fill out this form to contact WCG with any questions and comments, to learn more about our authors and insights into the 2025 Trends and Insights Report.