The following Insight is a featured article from WCG’s 2025 Trends & Insights Report. If you would like to read more insights from this report, please click here.
The integration of artificial intelligence (AI) and machine learning (ML) into clinical research is rooted in the exponential growth of data availability. In recent years, clinical trials have been bolstered by the collection of vast and diverse data sets, encompassing electronic health records, genomic sequences, real-world evidence, and patient-reported outcomes. For example, the volume of healthcare data is expected to reach 10k exabytes by 2025,1 providing an unprecedented reservoir of information for AI and ML applications. Indeed, AI algorithms have already demonstrated their ability to analyze this data with remarkable speed and accuracy, identifying patterns and insights that were previously unattainable.
Moreover, robust datasets are now enabling researchers to leverage AI and ML for predictive modeling with higher precision. For instance, a study published in Nature highlighted how AI models, trained on extensive genomic and clinical data, significantly improved the prediction of treatment responses in oncology patients. This leap in predictive capabilities underscores the transformative impact of high-quality data and advanced analytics in clinical research.
In discovery, ML models — both traditional and deep learning — are being employed to identify drug targets and predict molecular interactions that alter disease mechanisms. On the development side, ML applications are expanding rapidly. These include predicting trial success rates, optimizing protocols, selecting countries and sites, and even forecasting costs. Achieving these outcomes demands a structured data framework, defined modeling approaches, and cross-functional collaboration to interpret and implement model insights effectively.
Data-related challenges remain a significant hurdle. High-quality, structured, and accessible data is essential for reliable ML models. Companies must address issues like data governance, literacy, and security while fostering collaboration between business and technology teams. The expertise of business professionals is particularly critical to guide model design, select input variables, and interpret results, ensuring AI applications meet real-world needs.
WCG has been at the forefront of tackling these challenges, leveraging central benchmarking databases to enhance ML applications. By curating anonymized, high-quality data from client contributions and WCG’s proprietary resources, these centralized databases create an optimal environment for training and applying ML models. For example, WCG KMR’s benchmarking data serves as a unique peer resource, supporting companies as they build data-driven solutions to improve trial outcomes. This collaborative model demonstrates how shared data can elevate the reliability and effectiveness of ML in clinical research.
In a recent WCG webinar on DEI analytics, gradient boosting ML models were showcased to predict a site’s likelihood of enrolling diverse populations. By aligning data sources such as site enrollment figures, location demographics, social determinants of health, and disease prevalence, the models revealed actionable trends. For example, sites in areas with higher proportions of specific populations, like Asian and Hispanic communities, showed increased probabilities of enrolling those demographics.
Site type (institutional vs. community) was a key factor for enrolling Black participants in oncology studies. Additionally, social determinants like frequent doctor visits and higher use of preventive screenings were associated with greater diversity in enrollment. These insights help refine site selection and enhance DEI in clinical trials.
In 2025, the integration of AI and ML in clinical development represents not just an emerging trend but a pivotal shift. By focusing on high-quality data, fostering collaboration, and addressing data challenges, organizations can unlock the full potential of these tools. Strategic implementation of ML can accelerate trial efficiency, support inclusive research, and position the industry for a more innovative and equitable future.
References:
- LEK Consulting. “Tapping New Potential: Realising Value from Data in the Healthcare Sector.” https://www.lek.com/insights/hea/eu/ei/tapping-new-potential-realising-value-data-healthcare-sector.
Related Insights:
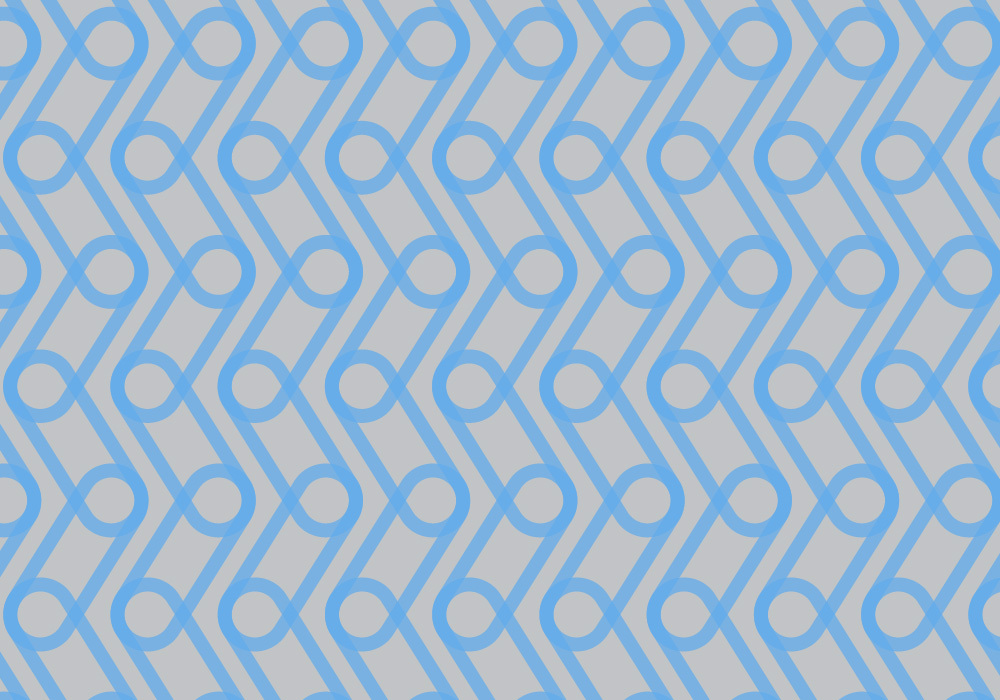
Ethical Review & AI in Clinical Trials
PodcastsLearn more about our Trends and Insights Report for 2025
Fill out this form to contact WCG with any questions and comments, to learn more about our authors and insights into the 2025 Trends and Insights Report.